An Empirical Approach to Dimensionality Reduction and the Study of Patterns

Dimensionality reduction is a technique that can be used to reduce the number of features in a data set while preserving as much information as possible. This can be useful for a variety of reasons, such as improving the performance of machine learning algorithms, making data more interpretable, and visualizing data in a lower-dimensional space.
5 out of 5
Language | : | English |
File size | : | 7074 KB |
Text-to-Speech | : | Enabled |
Print length | : | 325 pages |
Lending | : | Enabled |
There are a variety of different dimensionality reduction techniques available, each with its own strengths and weaknesses. In this article, we will discuss one of the most popular dimensionality reduction techniques, principal component analysis (PCA),and provide an empirical example of how it can be used to reduce the dimensionality of a data set and study patterns in the data.
Principal Component Analysis (PCA)
PCA is a linear transformation that can be used to reduce the dimensionality of a data set. It works by finding a set of new features that are linear combinations of the original features, and that capture as much of the variance in the data as possible. The first principal component is the linear combination of the original features that captures the most variance, the second principal component is the linear combination of the original features that captures the second most variance, and so on.
PCA can be used for a variety of purposes, including:
- Improving the performance of machine learning algorithms
- Making data more interpretable
- Visualizing data in a lower-dimensional space
An Empirical Example
In this section, we will provide an empirical example of how PCA can be used to reduce the dimensionality of a data set and study patterns in the data.
We will use the Iris data set, which is a collection of data on the sepal length, sepal width, petal length, and petal width of 150 iris flowers. We will use PCA to reduce the dimensionality of the data set to two dimensions, and then we will plot the data points in the two-dimensional space.
The following code shows how to perform PCA on the Iris data set using the scikit-learn library:
python from sklearn.decomposition import PCA
# Load the Iris data set iris = datasets.load_iris()
# Create a PCA object pca = PCA(n_components=2)
# Fit the PCA object to the data pca.fit(iris.data)
# Transform the data using the PCA object iris_pca = pca.transform(iris.data)
# Plot the data points in the two-dimensional space plt.scatter(iris_pca[:, 0], iris_pca[:, 1], c=iris.target) plt.show()
The output of the code is a plot of the data points in the two-dimensional space. The plot shows that the data points are clustered into three groups, which correspond to the three different species of iris flowers. This suggests that the first two principal components capture the most important patterns in the data set.
PCA is a powerful dimensionality reduction technique that can be used to improve the performance of machine learning algorithms, make data more interpretable, and visualize data in a lower-dimensional space. In this article, we provided an empirical example of how PCA can be used to reduce the dimensionality of a data set and study patterns in the data.
We encourage you to experiment with PCA and other dimensionality reduction techniques to see how they can help you improve your machine learning projects.
References
[1] Jolliffe, I. T. (2002). Principal component analysis. John Wiley & Sons. [2] Hastie, T., Tibshirani, R., & Friedman, J. (2009). The elements of statistical learning. Springer Science & Business Media.
5 out of 5
Language | : | English |
File size | : | 7074 KB |
Text-to-Speech | : | Enabled |
Print length | : | 325 pages |
Lending | : | Enabled |
Do you want to contribute by writing guest posts on this blog?
Please contact us and send us a resume of previous articles that you have written.
Book
Page
Chapter
Story
Library
Paperback
Newspaper
Shelf
Glossary
Bibliography
Synopsis
Manuscript
Scroll
Classics
Library card
Narrative
Biography
Autobiography
Memoir
Encyclopedia
Narrator
Character
Resolution
Librarian
Catalog
Card Catalog
Archives
Periodicals
Research
Lending
Reserve
Academic
Special Collections
Literacy
Thesis
Dissertation
Storytelling
Awards
Reading List
Book Club
Fleur Hitchcock
Carole Anne Carr
Walter Daelemans
Jim Ruland
Ralph Van Deman Magoffin
Stan Lee
Lynette Noni
Tea Krulos
Joos Korstanje
D Sundararajan
Vicar Sayeedi
Kimberly Willis Holt
Michael Fry
Haywood Smith
Rich Bradwell
Arthur Rackham
Elizabeth Partridge
Mick Finlay
Karinne Piat
Intisar Khanani
Light bulbAdvertise smarter! Our strategic ad space ensures maximum exposure. Reserve your spot today!
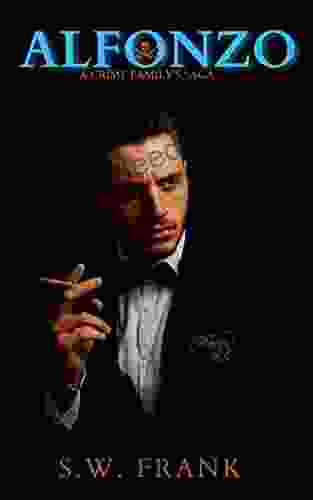

- Gabriel HayesFollow ·11k
- Patrick HayesFollow ·12.5k
- Douglas PowellFollow ·18.7k
- Jacques BellFollow ·16.5k
- Jacob FosterFollow ·7.8k
- Leon FosterFollow ·17.5k
- George BellFollow ·8.2k
- Jerome BlairFollow ·6.3k
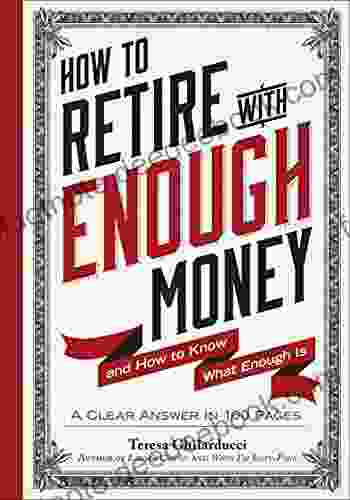

Unveiling the True Meaning of Enough: A Comprehensive...
: In the relentless pursuit of progress and...
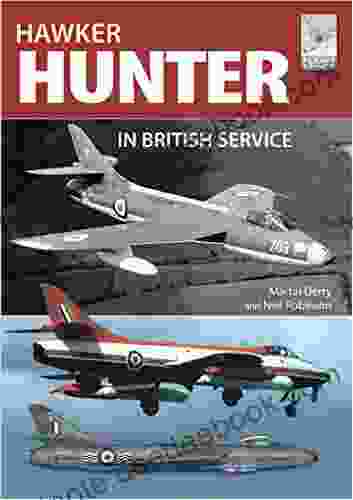

Hawker Hunter: The Jet Fighter that Shaped British...
Nestled in the halls of aviation...
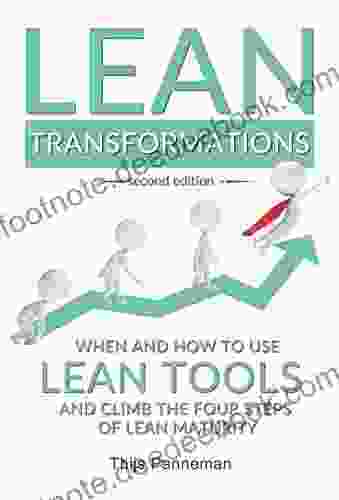

When and How to Use Lean Tools and Climb the Four Steps...
Lean is a management...
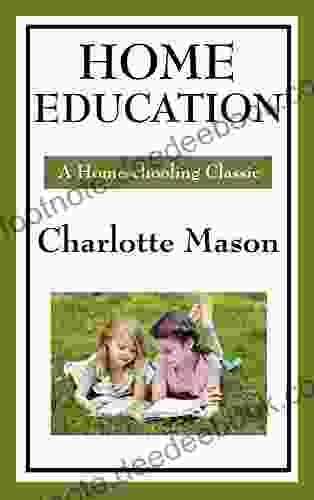

Volume of Charlotte Mason Original Homeschooling: A...
Charlotte Mason's original...
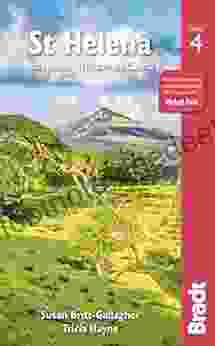

Ascending Tristan da Cunha: A Comprehensive Guide to...
Prepare yourself for an extraordinary journey...
5 out of 5
Language | : | English |
File size | : | 7074 KB |
Text-to-Speech | : | Enabled |
Print length | : | 325 pages |
Lending | : | Enabled |